Leading SEO software offers a comprehensive solution for competitor analysis, providing businesses with the tools they need to stay ahead of the competition.
These tools are not just a bunch of isolated features, but rather a cohesive set of tools designed to give businesses a holistic view of the competitive landscape.
One of the key features of leading SEO software is the ability to track and monitor competitors' websites. This includes analyzing their keywords, backlinks, and other SEO metrics. This allows businesses to identify gaps in their own SEO strategy and make adjustments as needed. For example, if a competitor is consistently ranking well for certain keywords, businesses can use this information to identify and target those keywords for their own website.
One of the major fallacies of most SEO software today is that the highest ranking site is the site that is ranking the highest in every search engine algorithm. This couldn't be further from the truth. Think of the top ranking sites as the ones that are the most consistent performers across all algorithms. The top SEO software platforms will be able to isolate the outperformers for each algorithm so your team can chase the right goal.
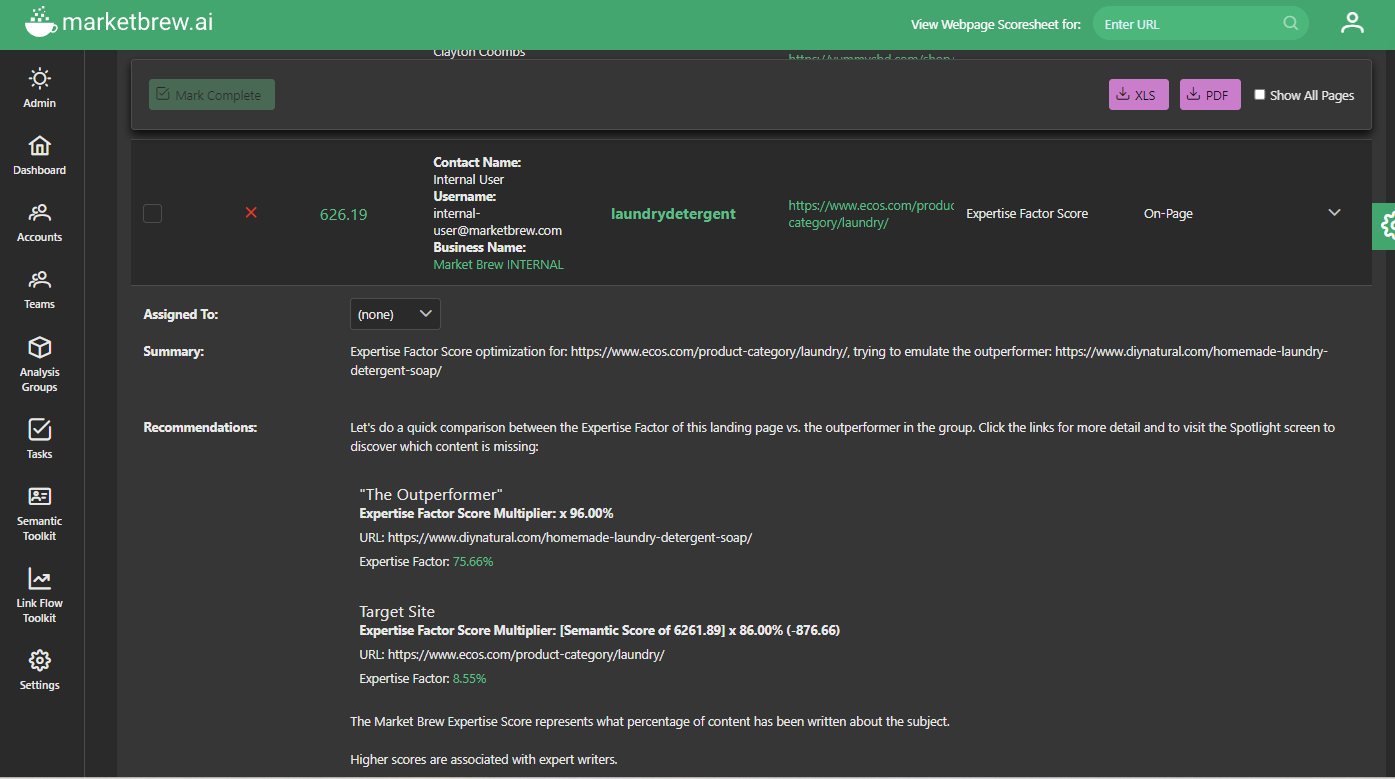
Leading SEO software also offers tools for analyzing the backlinks of competitors. This includes identifying the sources of their backlinks and the types of content that are attracting links. This information can be used to improve the quality and relevance of a business's own content, as well as to identify potential link building opportunities.
In order to provide the most accurate data, leading SEO software should analyze data at the first principles level. For example, the data granularity should be at the individual link level, with the ability to determine the link equity based on calibrated link algorithms. This first principles approach creates an SEO software that is able to provide an accurate picture of things like a true link graph, which is the cornerstone of any search engine.
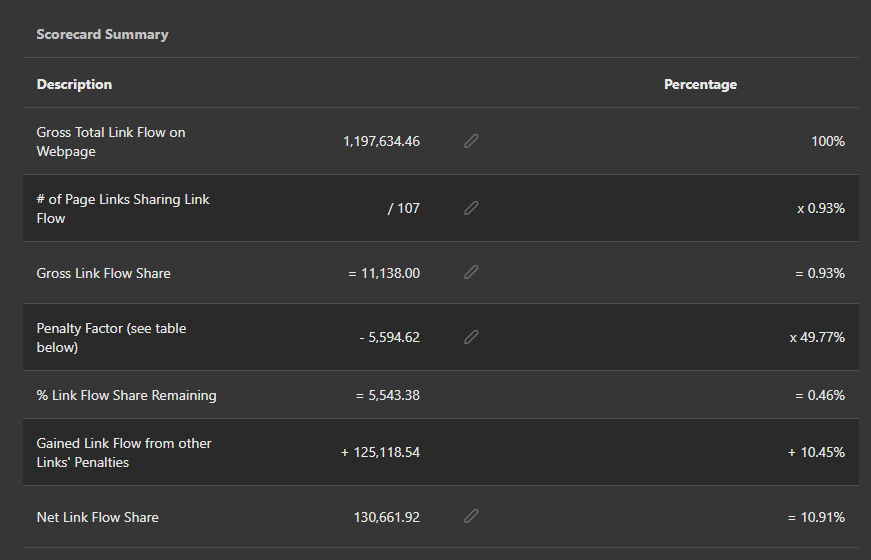
Overall, leading SEO software offers a comprehensive set of tools for competitor analysis that goes beyond a bunch of isolated features. These tools work together to give businesses a holistic view of the competitive landscape and provide actionable insights that can be used to improve their own SEO strategy.
By using these tools, businesses can stay ahead of the competition and achieve better results from their SEO efforts.